In a recent paper by the International Association of Crime Analysts (IACA), social network analysis (SNA) has proven to be a useful and important tool in crime analysis. More specifically, it helps law enforcement to better understand criminal networks, co-offending patterns, victimization, drug trafficking networks, as well as many more areas. The value in applying network analysis to crime lies in the main idea of identifying who a person of interest associates with, how strong these associations are and attempt to predict future actions of these POIs.
One specific idea this paper discusses is Mark Granovetter’s concepts of strong and weak ties between two nodes, or in this case between two people. For example, strong ties could represent family and close friend relationships while weak ties could represent acquaintances. In particular, the concept of the “forbidden triad” (Figure 2) is especially relevant to crime analysis. This concept of the “forbidden triad” is essentially the same concept as strong triadic closure that is discussed in CSCC46 material, which is the concept that asserts that if a node A shares a strong tie with node B and A also shares a strong tie with node C, then B and C should share at least a weak tie (B and C should share a weak or strong). In a real-life social sense, if these nodes were people, then persons B and C should at least have an awareness of each other. As described in this paper, “if John (A) is a suspect in a crime, it may be that while Bob (B) may have more information to share about John, Tim (C) might be more willing to share the limited (but potentially very useful) information he has with detectives assigned to the case.”
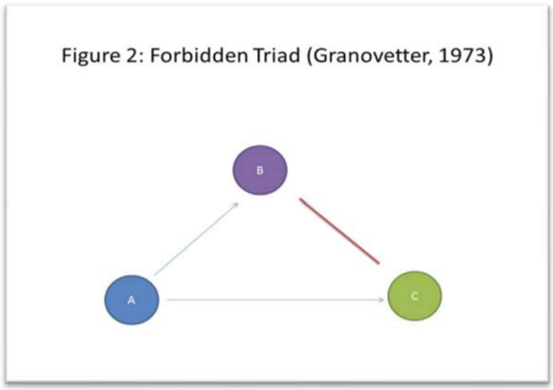
Another example of information that can be uncovered through applying network analysis to crime networks is the identification of “key players.” In an analysis of a given crime network, the visual output would resemble a graph (Figure 3), displaying nodes representing either individual persons or larger groups or entities and edges between these nodes representing an association between two persons or groups. From simple inspection and from further mathematical analysis (e.g. betweenness, closeness centrality) it can be concluded that nodes E and G play important parts in the flow of information in this network. The paper also explains that one of the most common network strategies to detect these key players centers around the concept of betweenness, which is an idea that has also been discussed in CSCC46 course material.
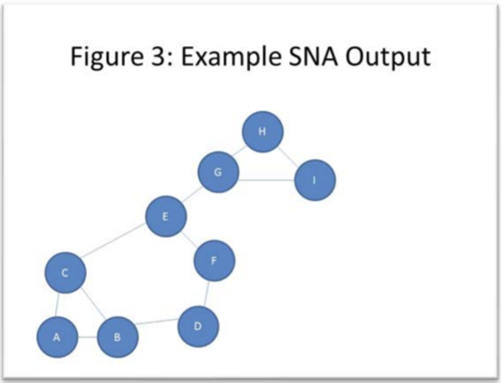
One case example of social network analysis having a positive impact on crime analysis involves the Richmond, Virginia Police Department searching for a homicide suspect for approximately one month, at which point their crime analysis department decided to construct a network analysis of the suspect’s social network. From this analysis, they identified key persons and quickly notified them that the suspect was wanted and were told to tell police if they would be in contact with the suspect. It turned out that because of the police contact, the suspect turned themselves in within hours. The paper explains that in this scenario, social network analysis assisted law enforcement in identifying key persons related to the suspect beyond just family members and close friends.