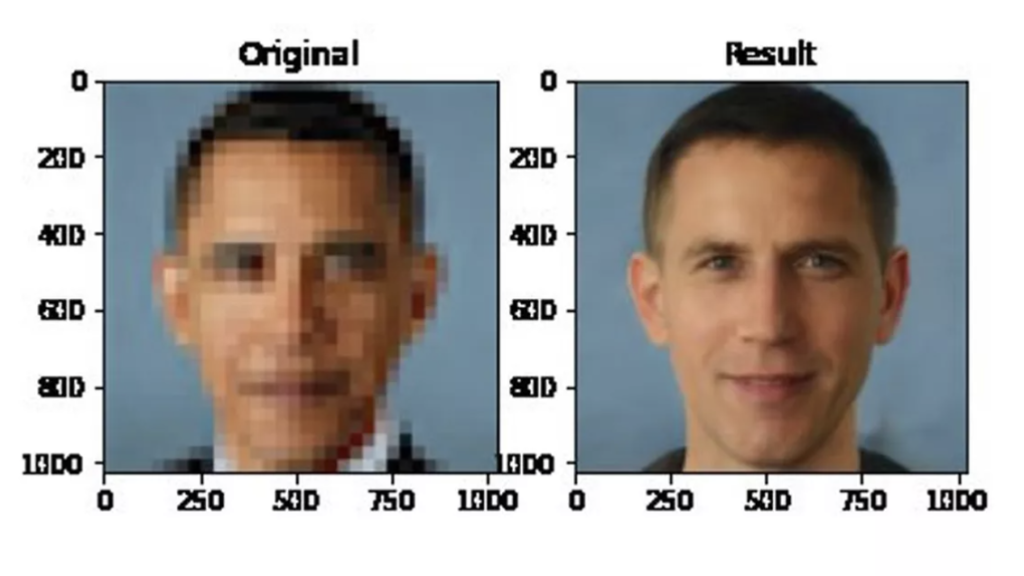
Generative adversarial network (GAN) is an exciting recent innovation in machine learning. “Given a training set, this technique learns to generate new data with the same statistics as the training set. For example, a GAN trained on photographs can generate new photographs that look at least superficially authentic to human observers, having many realistic characteristics.” (Wiki)

The algorithm of GAN can be understood as a “Minimax Zero-Sum Non-Cooperative Game” that two neural networks, generative network, and discriminative network, are contesting against each other in the game. The generative model is trained to produce authentic images to fool the discriminator, and the discriminator is trained to distinguish between fake images produced by the generative model and the real images.
Researchers found that it is difficult to train GAN as the two networks cannot reach the optimal at the same time. This phenomenon can be explained by why it is difficult to find the Nash Equilibrium using gradient descent.
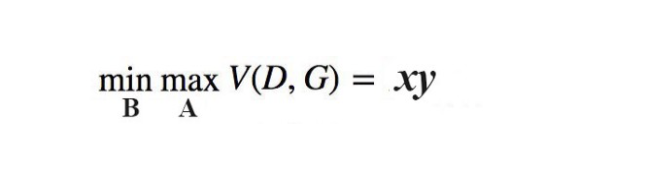
Consider a Minimax game with two players A and B, which control the value of x and y, respectively. Player A wants to maximize the value xy while B wants to minimize it. Analytically, we know that the equilibrium reaches when x=0 or y=0.
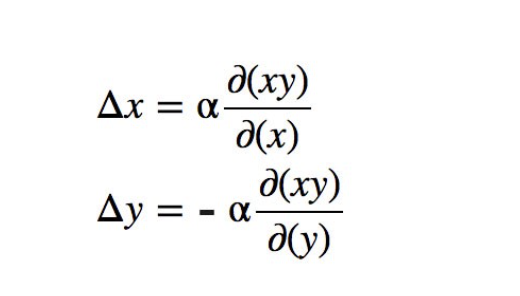
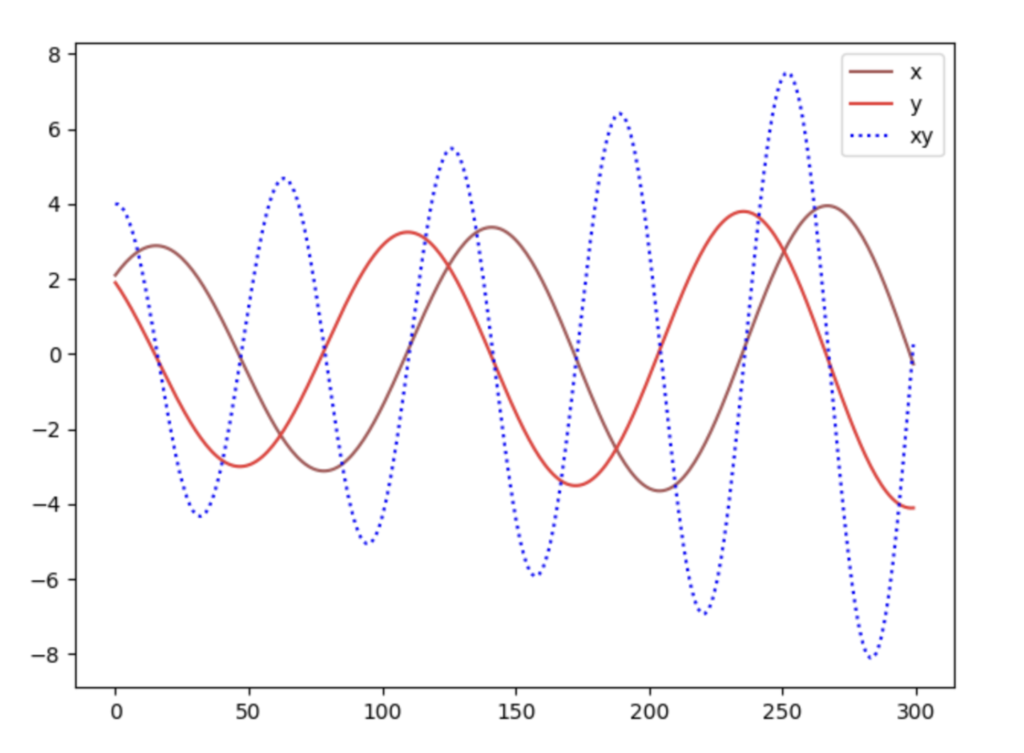
However, if we update the parameter x and y based on the gradient of the value function V, from figure 5, we find x and y are oscillating around 0 and do not converge. Hence, gradient descent has flaws to find the Nash equilibrium.
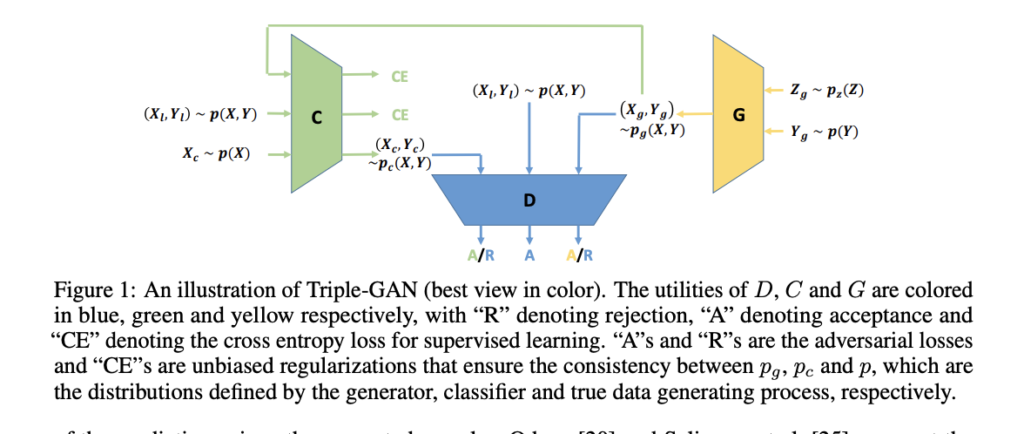
Researchers have also found it is often the case that the discriminator can reach near-optimal, and the generator is unable to model the distribution of the true data. Triple-GAN was proposed to improve the performance of the generator by introducing a third player, classifier. The utilities of the generator and discriminator differ from the ones in GAN slightly. The generator and the classifier characterize the conditional distributions between images and labels, and the discriminator solely focuses on identifying fake image-label pairs. The authors of the paper proved that when the class conditional distribution between the classifier and the generator becomes close, the generator and classifier can nearly model the true data distribution. Hence, Triple-GAN introduces a term RL that penalizes the loss function if the class conditional distribution between the classifier and the generator diverges too much.

Now, take a look at how Triple-GAN has reshaped the dynamics of the game. The generator and classifier are trained to fool the discriminator, and the discriminator is trained to distinguish fake image-label pairs. But this time, the cooperative characteristic is introduced to the game. As mentioned in the paragraph above, the loss function is penalized if the class conditional distribution between classifier and generator diverges too much. In other words, the classifier and generator lose points if they have the same class distribution. And thanks to the cooperation with the classifier , the generator is able to choose a better strategy for itself and can model the true distribution data more closely.
References:
1: https://arxiv.org/pdf/1703.02291.pdf
2:https://arxiv.org/pdf/1406.2661.pdf
4:https://en.wikipedia.org/wiki/Generative_adversarial_network