In this class we have seen how graph analysis can be applied to many different topics of study, some expected and many surprising. A paper published by Martin and collaborators showcases yet another field where graphs can be of help: dream analysis.
The study of dreams can reveal early indicators for mental health disorders, such as schizophrenia or psychosis. They can also give us information about how the brain is functioning during sleep. Our brains undergo different phases of activity during sleep, and the nature of the dreams generated can inform our knowledge of the underlying processes in different phases.
Martin et al. (2020) studied dreams generated during REM and non-REM cycles. Common (non-graph related) ways to evaluate dreams include: recall rate – whether the participants remember the dreams at all, report length – such as the number of unique or total words, or narrative complexity – the story-like organization of the dream as evaluated by external judges according to a defined ratings scale. It is for this last item where Martin et al. employed the use of graphs.
The raw data collected was dream reports, transcriptions of the participants recounting their dreams. To create a graph from a report, each unique word formed a node and edges were drawn between words that appeared consecutively in the report, such as in the figure below.
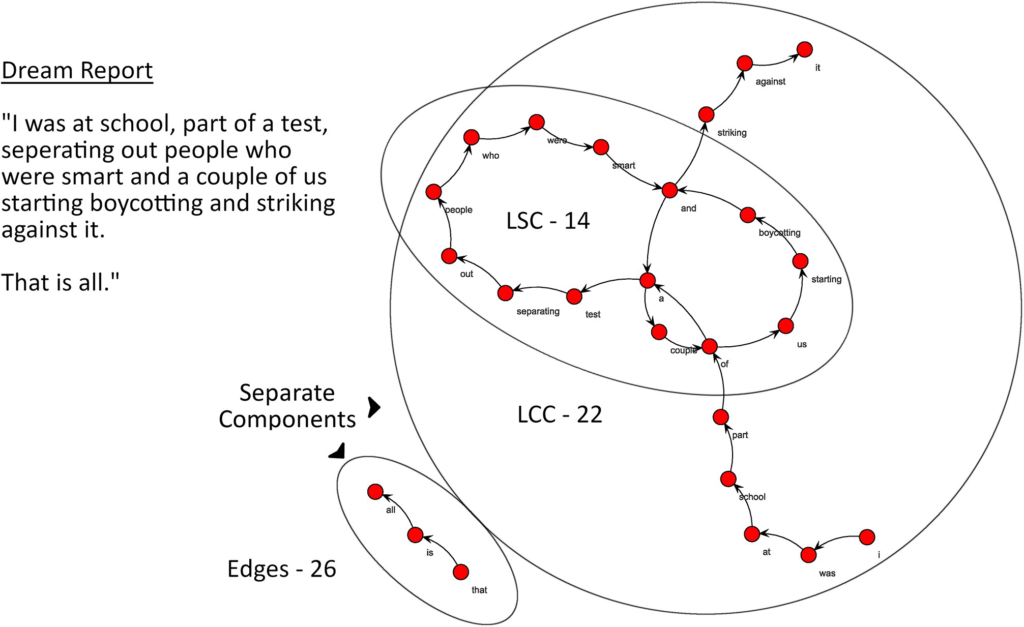
From these graphs, they analyzed the size of the largest connected component (LCC), size of largest strongly connected component (LSC), and random-likeness (resemblance to graphs generated by random chance). They found that larger LCCs and LSCs were indicative of more complex dream reports. Lower random-likeness was also correlated with higher dream complexity. We can see that more connections between separate elements of the story and more unlikely story structure, elements that can make a narrative more complex, is captured in the graph analysis of the dream reports.
The methodology employed in this study was an interesting example of how graph analysis can be applied to data that is qualitative, especially where the relationship between the nodes seems almost arbitrary (order of the words). The complexity of a narrative is a very abstract concept that even with human judgement is difficult to analyze; this methodology lends a new meaning to “a picture is worth a thousand words”.
Source: https://journals.plos.org/plosone/article?id=10.1371/journal.pone.0228903