In lecture we have looked at PageRank through the ‘Flow Model’. Using this model, we were able to apply the Scaled PageRank algorithm to produce the equilibrium values indicating the best nodes to use in the graph. However, what happens when we apply this idea to sports?
In August 2020, Ryan Jones asked this question and decided to try to predict the 2020 Stanley Cup winner based on win/loss data throughout the season. Mr. Jones recognized that the best team could loosely be defined as the team who has beaten the most (unique) teams. This is because if a team was able to defeat all other teams in the league, although it’s not certain that they’ll win in a 7-game playoffs series, it shows that they have the potential to beat every other team, given that team rosters don’t change much throughout the season.
To set up this analysis, Mr. Jones created a directed graph where every team in the NHL was a node, and if team A lost to team B during the 2019-2020 season, team A would create an edge to team B. One interesting point to this setup is that it gauges the quality of wins. This can be done since using the ‘flow’ model, if a team is one of the worst, they will have many out-edges meaning that each node receiving those edges will receive less flow. Consequently, if a team is one of the best, they will have less out-edges, indicating that every team receiving flow from this team (one of the best teams), will receive more flow from this team than one of the worst teams, since the win with the better team is more meaningful than beating one of the worst teams.
Let us look at the data and how Mr. Jones’ prediction lined up with the actual 2020 NHL Playoffs:
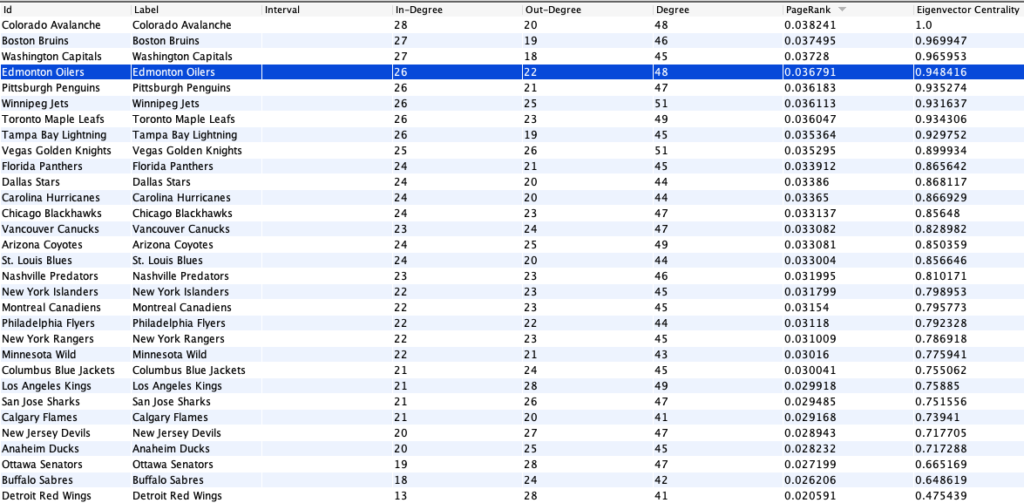
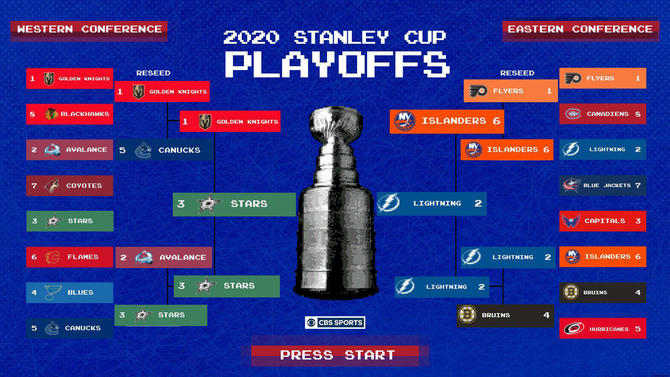
Looking at Mr. Jones data, we can see that Colorado, Boston, Washington, and Edmonton were the top 4 teams as they had the highest PageRank among teams in the NHL. However, Colorado and Boston were the only two teams of the four who made it to round 2 of the playoffs, and both teams lost in round 2. Whereas Tampa Bay and Dallas both made it to the finals even though their page rank was not even in the top 7. Using this information, we can conclude that at least in hockey, PageRank is not a very strong indicator of the team who will win the Stanley Cup. There are many factors which could have affected this outcome such as COVID effects on the league, randomness of performance, and veteran players who have more experience in playoff settings.
I found this article very interesting as it explores PageRank in an unconventional context, Hockey! Intuitively Hockey does not have much of a correlation with PageRank, however Mr. Jones was able to tweak the perspective of the data so that it would reflect a PageRank scenario yielding equilibrium values. Although the outcome did not strongly reflect the prediction from the data, it still makes a case for why certain teams have a better chance of winning the Stanley Cup. For example, it is very unlikely that the team with least amount of wins against unique teams will win the cup, not only because they have a lower win/loss ratio, but because if they have lost to teams during the regular season, what evidence points to them winning against those teams again in the Playoffs?
Perhaps there are certain pieces of data which can sharpen the accuracy of the PageRank used in the prediction of sports, and thankfully we will never run out of new sports data to keep trying out!
Source:
https://www.searchenginejournal.com/pagerank-predict-nhl-playoffs/375125/