During the covid-19 pandemic, many of us stay home for self quarantine. Among many entertainment choices, a classic mafia style online game called Among Us has topped the charts for the current most played game in 2020. The mafia style game follows the premise of crewmates who are trying to repair the spaceship while imposters pretending to be crewmates slowly eliminate the crewmates before the ship is ready to make their escape. In this game, there exists dominant strategies and payoff matrices for the decisions and actions of crewmates and imposters.
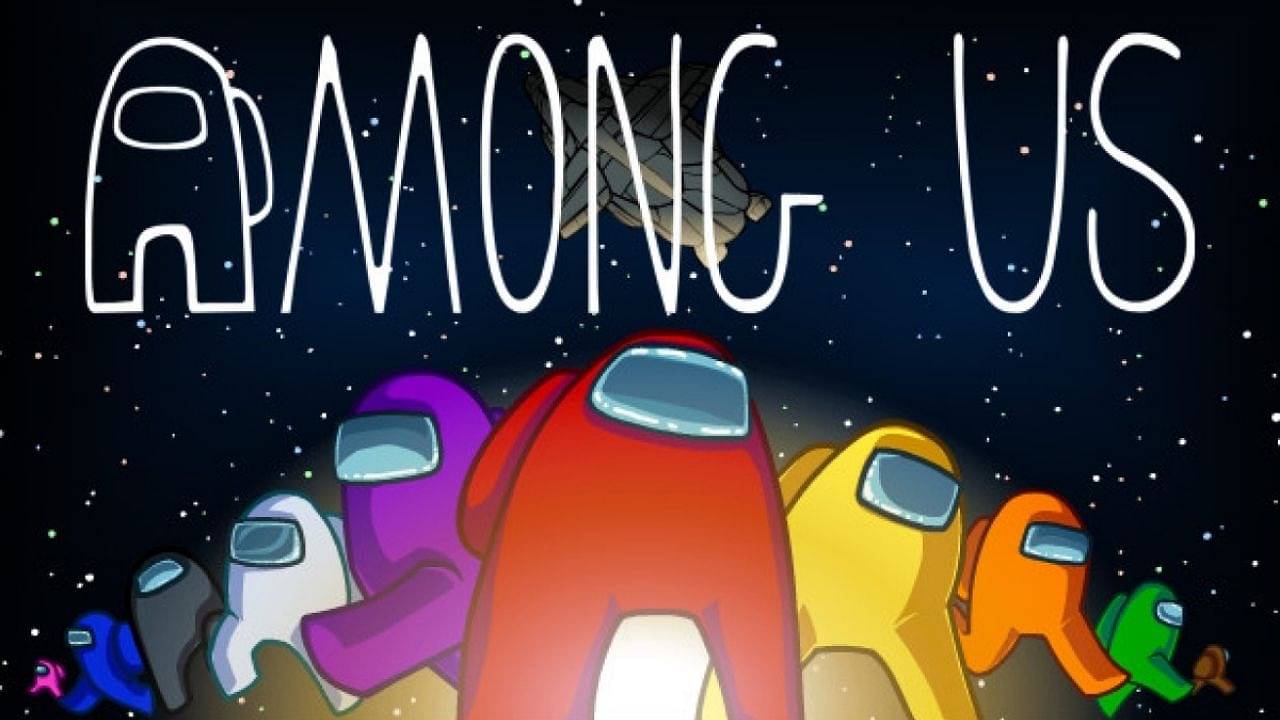
First off, we can examine the utility functions of crewmates who want to perform as many repair tasks around the ship while staying alive. One can argue that different players will value staying alive or speeding through tasks to be more important on an individual basis. For this a common choice crewmates handle are to either stay together in a group, or run off alone to your own tasks. Next we evaluate the perspective of the imposters. Imposters want to eliminate crewmates without being noticed, so crewmates sticking together is bad for them, while crewmates running off alone makes for an easy job. As such, we can relate to game theory and arrive at a payoff matrix that may resemble something like this:

One may find that sticking together is the dominant strategy, yet why is this game not always won by crewmate who possess a dominant strategy against the imposters that do not? The reason may be very well an incentive to cheat among the crewmates. The reasoning being: if others are sticking together, then me running off would let me do repairs faster and not be at risk of getting eliminated by an imposter within the group. Thus another matrix is between crewmates may resemble as follows:

Fortunately for the imposters, they get a chance at always catching someone running off solo because the incentive to cheat a huge cluster brings the Nash equilibrium to scatter large groups. This is in fact the normal behavior of average games where players sometimes only forms small partnerships and travel together as a compromise to both extremes (neither group nor solo). This risks whether or not we trust the partnership on predetermined roles. They can either be an imposter marinating a crewmate for a solid alibi, or they can be crewmates looking for protection:

In this event, the column chosen is pre-determined, but we won’t know for sure until it’s too late, making intuition a large portion of the decision making process. Trusting someone who happens to be a crewmate is mutually beneficial, while trusting an imposter could get you eliminated, or even becoming a “third imposter”, someone who spreads misinformation in favor of imposters. This concludes the main decision making aspect of the game where dominant strategies conflict with an incentive to cheat. Do you feel its better to stick together, or would you rather trust nobody?
Sources:
https://medium.com/@kaustubh.q/among-us-and-game-theory-f74c8ac9f05
https://www.theguardian.com/games/2020/sep/29/among-us-the-ultimate-party-game-of-the-covid-era
https://thesportsrush.com/among-us-downloads-among-us-tops-downloads-in-october-2020/