What is happiness?
According to the 2020 World Happiness Report, Finland is one of the happiest countries. The report collects its data by surveying six variables to measure people’s general satisfaction with their life. These variables are healthy life expectancy, freedom, generosity, GDP per capita, social support, and absence of corruption. The report’s definition of happiness is scientifically interchangeable with Subjective Well-Being (SWB), which measures the satisfaction of one’s life. However, happiness is an emotion that humans can experience subjectively in many different ways. Therefore, the question follows “what do people organically relate to the feeling of happiness?”
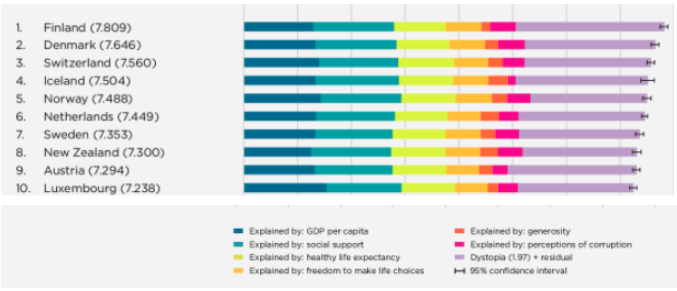
People share a vast majority of their feelings and life experiences on social media via posts. On platforms such as Twitter and Instagram, users classify posts by the use of hashtags. Hashtags are metadata used to help users more easily discover content. Hashtags can provide concise context to posts about what the users were feeling, or experiencing at the time of making their post. By using the material taught during the first few weeks of CSCC46, we can leverage graphs to extrapolate information from hashtags about what people relate to happiness.
Graphs, constructed with vertices and edges, are a mathematical representation of networks. In our situation, we want to represent a network of hashtags. In such a graph, hashtags would represent vertices because they denote data points we want to analyze. Intuitively, an undirected edge connecting two vertices represents an occurrence (post) using both hashtags. To discover the organic meaning of happiness on social media, we analyze the topics (hashtags) users relate with ‘#happiness’ using our undirected graph structure. Additionally, analyzing hashtag relationships to each other can be used to develop measures of happiness.
Calculating the betweenness of nodes in our undirected-graph identifies the importance of specific hashtags in the network. The betweenness calculations can help detect how hashtags cluster together to form communities in the happiness network, such as in the Girvan-Newman algorithm discussed in lecture. Hashtag communities in the happiness network can derive measures for happiness on social media. For example, the happiness network on Instagram has three measures:
- Experiences e.g. summer, travel, art, nature, … etc. (community 0)
- Feelings e.g. motivation, inspiration, success, … etc. (community 1)
- Celebration e.g. wedding, party, ceremony, … etc. (community 2)
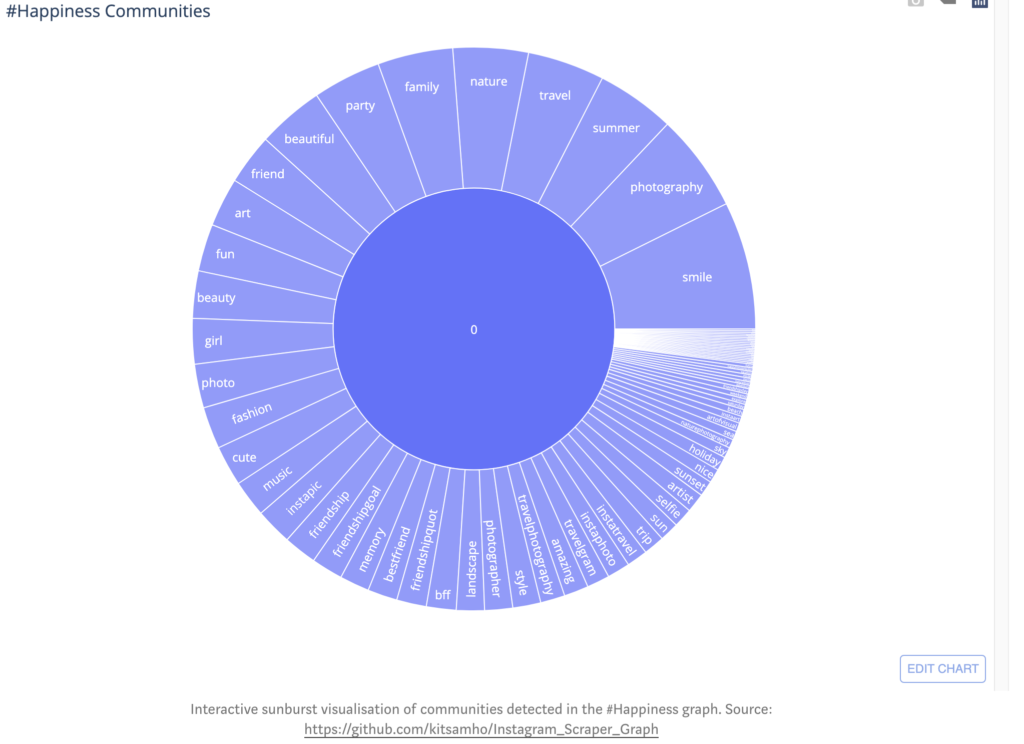
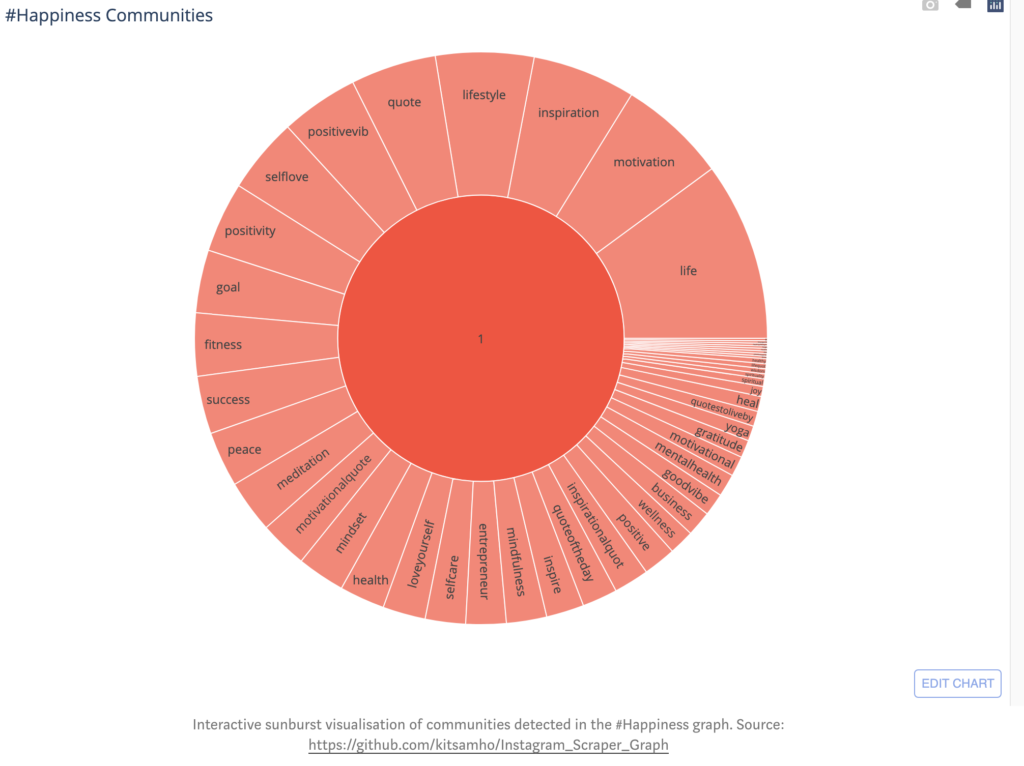
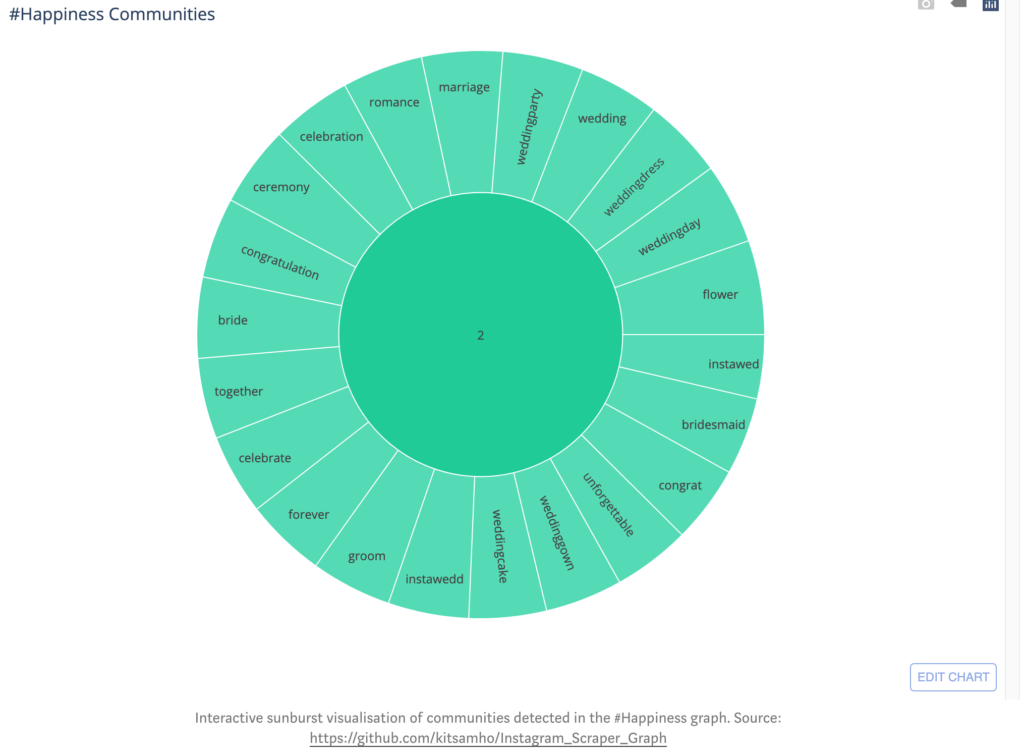
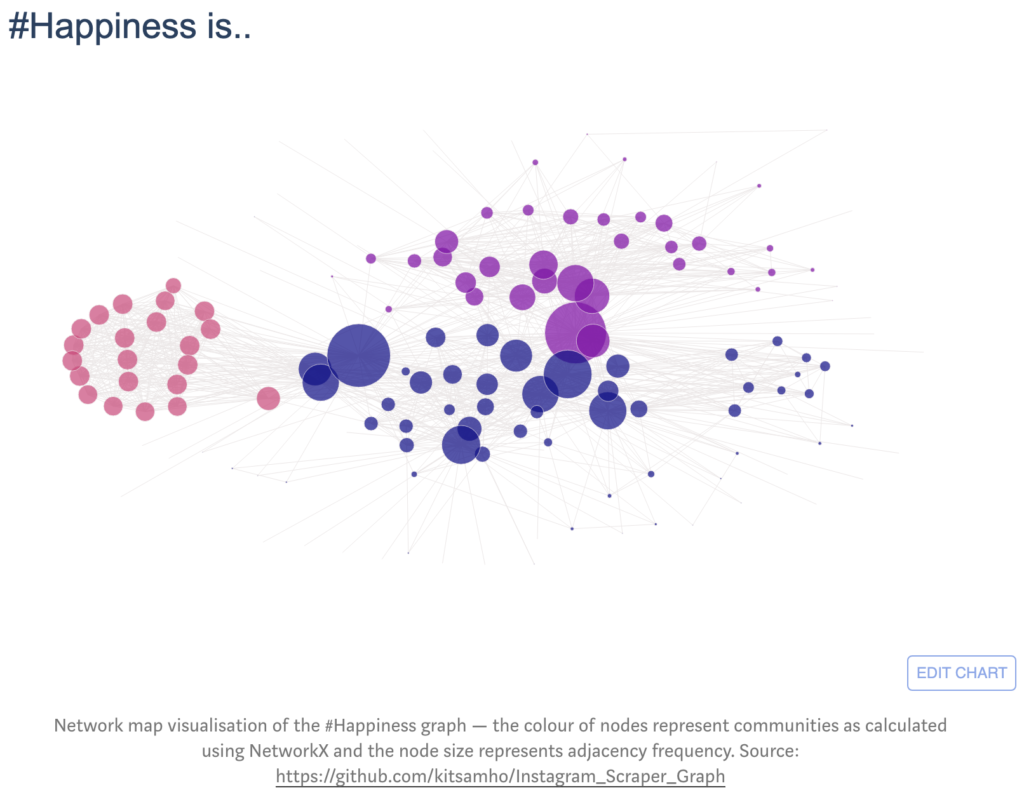
Other insights we can extrapolate from our undirected graph is the connectivity of nodes by calculating the degree to see how well-connected hashtags are in the happiness network. For example, nodes from the feelings community have a fairly uniform probability of edges between-one-another. Thus, they have somewhat equally likely to be used (felt) together when creating a post. Additionally, the local clustering coefficient of each node can inform the embeddedness of hashtags within communities in the happiness network. Furthermore, we can determine the meaningfulness of the clustering coefficient and degree properties in our happiness network by comparing it with the Erdös-Renyi Random Graph Model.
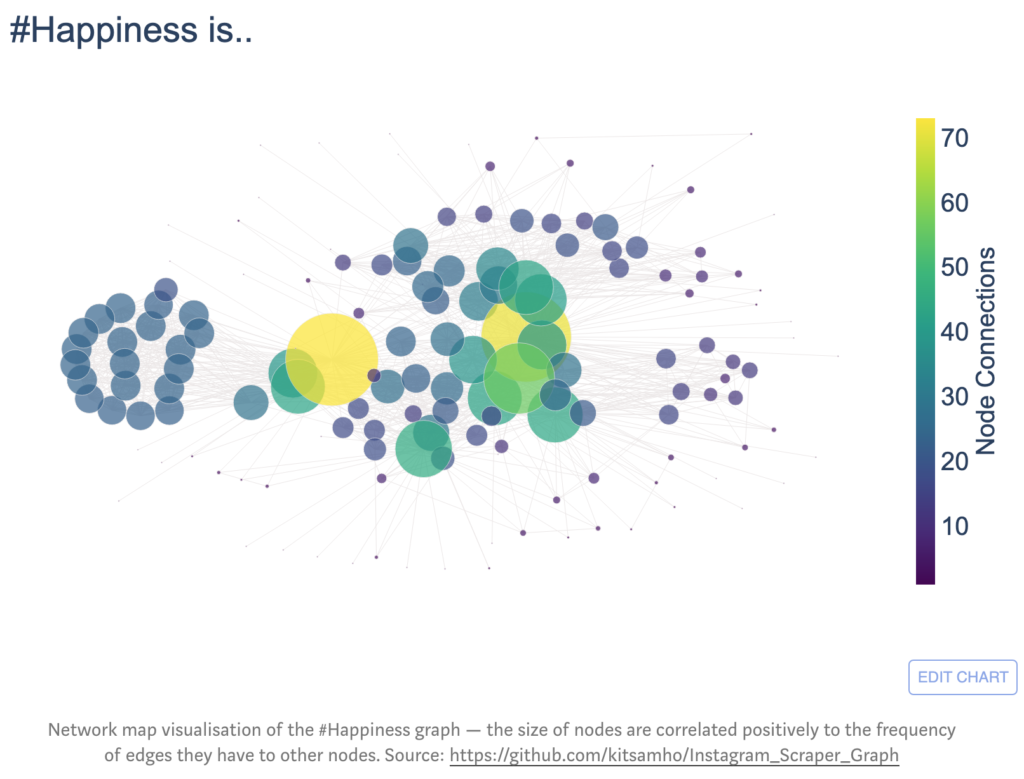
Limitations
The measure of what people relate to happiness based on social media posts is a novelty. The feelings shared on social media can’t be considered the absolute truth. People can over or under – exacerbate their feelings or experiences on social media. Additionally, some users on social media platforms may post more than others. Consequently, the data extrapolated from posts may only be representative of a few highly active users.
Other implications of hashtags and network analysis
Network analysis can not only provide insight to novel questions such as the meaning of happiness for people on social media, but also may provide insights and user sentiments about current political events, or trending products.
Graphs were created with code found here: https://github.com/kitsamho/Instagram_Scraper_Graph
Sources:
https://www.nytimes.com/2020/03/20/world/europe/world-happiness-report.html
https://worldhappiness.report/ed/2020/social-environments-for-world-happiness/
https://nobaproject.com/modules/happiness-the-science-of-subjective-well-being