Recently, you might have noticed that Twitter has modified its retweet feature in order to accommodate the influx of information that would emerge with the 2020 US election. Instead of being able to choose between a retweet and a quote retweet, Twitter now automatically prompts you to quote retweet in an effort to make you, the user, question whether the information you’re planning on retweeting is actually true and verify it instead of blindly spreading what might be misinformation. Why did Twitter release this feature at such an opportune moment and what might be the underlying factors that led to its creation?
Online platforms that either make use of search engines, have some form of a recommendation system or include a news feed (like the “News” section on Twitter’s trending page) rely heavily on ranking algorithms in order to determine each source’s popularity and decide what to show the user first as “top-ranked” sources. This ranking systematically affects the kind of information that gains traction among people regarding anything from products and services to even events and ideas. Although this may seem harmless on the surface, many users place a certain level of trust on these top-ranked results — regardless of whether or not they may be correct, lead to political polarization or reinforce certain judgement biases — purely because they are “top-ranked”.
Few-Get-Richer Effect
You’ve all heard of the “rich-get-richer” effect. For example, when items are ranked based on popularity, the popular ones are most likely to keep getting more popular. The popularity of a certain item isn’t always directly proportional to its quality, especially in settings like this that are characterized by these dynamics — the randomness of which may lead to ‘noise’ in the ranking, causing items that may not necessarily be of good quality to skyrocket within the rankings of a system. The “few-get-richer” effect takes this to a whole other level, by resulting in a systematic ranking bias: when two distinct classes of items are pitted against one another, items from the smaller class become better ranked than similar items belonging to the larger class.
There are two criteria necessary for the “few-get-richer” effect to emerge:
- a popularity ranking system where more clicks = higher rank
- the available items can be partitioned into two or more distinct classes
In addition, there are also two behavioural assumptions being made:
- users’ tendency to click on top-ranked items
- heterogeneous user preferences for the item classes (some prefer one class, some prefer the other and some are indifferent)
Cats vs. Dogs Experiment
Keeping all this in mind, an experiment was performed:
- Participants clicked on 1 out of 20 possible pictures, each of which belonging to either class M0 (cat pictures) or M1 (dog pictures)
- Effect of popularity-based ranking was measured by the total number of clicks on items that belonged to class M1
- Initial popularity of all the pictures was uniformly initialized to 1, but would then change dynamically as the experiment progressed
- Before partaking in the experiment, each participant was asked whether they were a “cat person”, “dog person” or “neither” of which:
- 30% were a “cat person”
- 55% were a “dog person”
- 15% were “neither”
They were then shown 20 photos with the message “Please click on a photo from the following list of photos of cats and dogs.” The photos were displayed in a vertical list with only 3 to 4 photos being visible immediately without having to scroll down. The order of the items displayed on this vertical list dynamically changed according to its popularity with the more popular ones showing up at the top and being more easily accessible. For the purposes of exploring the rank evolution of M1, the items in M1 always started at the bottom of the list.
Case 1: M1 = 2
In this case, there were only 2 dog pictures along with 18 cat pictures. It was found that despite having just 2 dog pictures, the total traffic attracted by items of class M1 was larger than that of those belonging to class M0 resulting in those 2 dog pictures to quickly climb up the ranks and maintain their high popularity throughout the experiment.
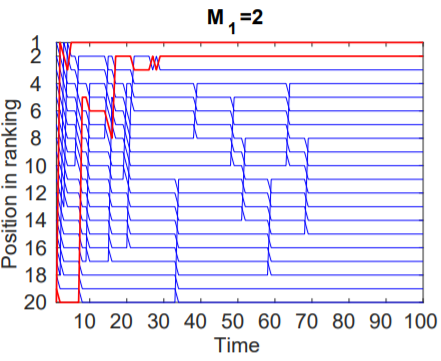
Case 2: M1 = 9
In this case, the number of dog pictures was equal to the number of cat pictures. The total traffic attracted by items of class M1 was similar to that of class M0 resulting in a ranking where the dog pictures were spread through the different popularity positions during the experiment.
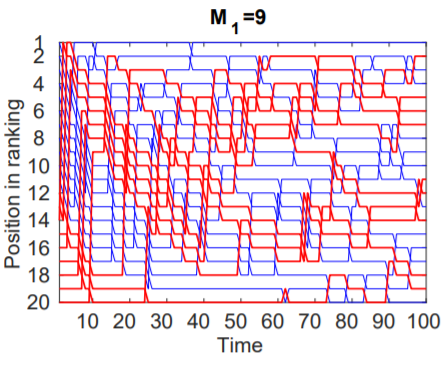
Case 3: M1 = 18
In this case, there were 18 dog pictures with only 2 cat pictures. Despite the large variety of dog pictures to choose from, the total traffic attracted by the items of class M1 was smaller than those belonging to class M0 resulting in the two cat pictures climbing to the top of the rankings while the remaining 18 dog pictures occupied the bottom ranks throughout the experiment.
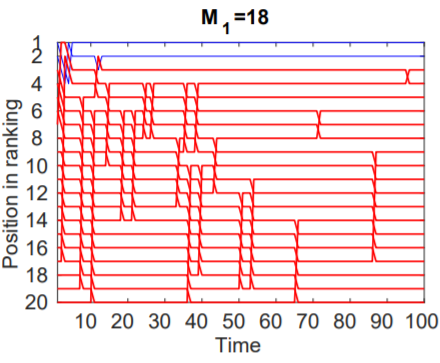
Conclusions
This experiment reached two conclusions regarding popularity-based ranking:
- it had a systematic effect on the traffic accumulated by items that were initially present at the bottom of the screen
- when there are fewer items belonging to a particular class, the total share of traffic attracted by this class becomes larger
The “few-get-richer” effect has its pros and cons regarding the quality of information people may obtain from online platforms that use ranking algorithms. On one hand, when there are a few relevant items, it allows them to become more accessible as they gain popularity. On the other hand, if the few items are irrelevant or ‘fake news’, the ranking serves as a catalyst for the misinformation they spread, especially when there may be a strong preference for it or no one reports it. A measure that can be taken to reduce the “few-get-richer” effect is to create a ranking algorithm that is independent of the number of items in each class.
So the next time you decide to get your daily dose of news or go through your Twitter trending feed, ask yourself whether the first results you see are necessarily the most credible ones, maybe try to scroll down and check out some of the lower options. After all, you may chance upon some diamonds the deeper you dig down and unlike in Minecraft, there’s no chance of falling into lava.
Source: Germano, F., Gómez, V., & Le Mens, G. (2019). The few-get-richer: A surprising consequence of popularity-based rankings? The World Wide Web Conference, 2764–2770. https://doi.org/10.1145/3308558.3313693