As seen in class, the Page Rank algorithm is an important algorithm for helping us organize the web. In fact, what might sound as one of the most mundane uses for any algorithm, the web’s equivalent of perhaps a librarian, figuring out relevant books from irrelevant and deciding which books go where, is quite the understatement. It is this very algorithm that helped form the roots of a very small, tiny company that you may have not heard of – Google. Yes, a household name and the company that engineers around the world dream of working at. The life of this company started from a simple question. “How can we structure the web?”. The answer to which was Page Rank.
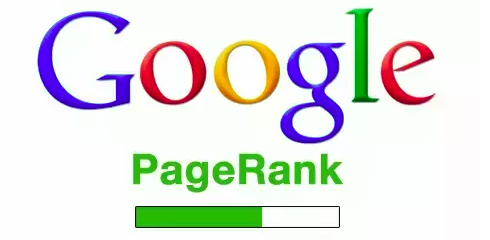
Ask anyone, regardless of all the services that Google provides it is no doubt that the cornerstone of the company is still its search engine. Its bread and butter is providing you everything you might need from the web based on only a few words or phrases. It will always do its best to provide the most relevant, and reputable web pages that will almost always leave you satisfied. In fact, it is quite rare any of us even have to go to the second page of search results. Is this magic? Larry Page and Sergey Brin, the founders of Google, would beg to differ. Designed by both in 1996, this algorithm used the very structure of the internet to assign a Page Rank value to each page in the graph structure of the web. It was this that Google search used to figure out which order the search results should appear in. However, Google’s search takes in up to 200 other factors as well to determine these rankings, which makes it clear why no other company has ever had as much success in the search engine game. Regardless of that, it is still no doubt that Page Rank is still the most famous and widely known algorithm out of all the ones that are used.
It was in the year 2000 that Google’s toolbar enabled users to not only search the web but to also have a look at the Page Rank of individual pages. However, this visibility often created competition and fraudulent uses of the algorithm with link selling and link farms. This was also one of the very interesting problems that we had a look at in our homework assignment where we saw how these metrics could be easily inflated with the use of very specific graph structures, and often even interlinking two farms that aided in further boosting these metrics. Here we see that the knowledge of the structure of the web as well as how the rankings are done not only make it clear how searching the web is conducted but also open the door to evildoers who seek to exploit it.
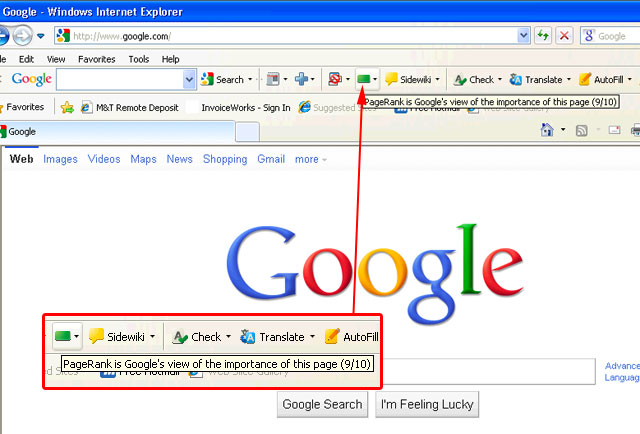
Further down the timeline, in 2003, Google’s attempts to fight back against link selling led it to take specific actions against a network known as SearchKing. The site itself and many of the pages on this network had their ranks penalized. SearchKing sued Google following this, however, Google came out victorious in the legal battle and pushed back against all kinds of link selling as well as other fraudulent means of trying to play the algorithm. This proved to not be effective, however, as no matter how much Google fought back, other link farms would open up. Google used a modification to the algorithm that made deductions to the ranks of detected and suspected link farms that inflated the rankings and manipulated search results. Thus, the rankings for these would be deflated and would not appear in the search results.
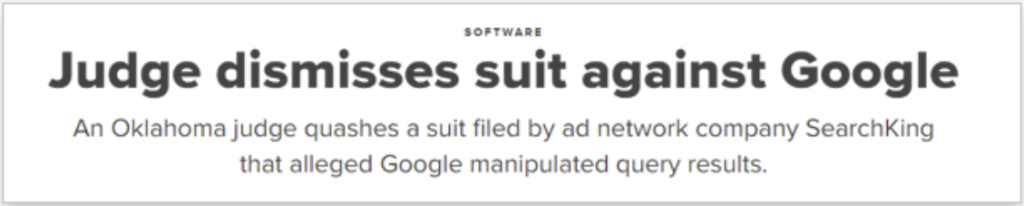
Starting in 2008, Google realized that although Page Rank on its own was a successful and effective way of organizing the web, these bad players made it difficult to keep search results up to the high quality that people expected from them. Thus, Google went dark with its Page Rank algorithm. This simple and elegant solution to the internet itself was not quite enough to be the trillion-dollar idea it is today. Google needed to modify it and grow it into something that could not easily be tampered with. Chrome was released soon after and all the toolbars that had lived on people’s internet browsers for so many years slowly died and disappeared after their long lives of service. As long as people knew the algorithm, it was open to manipulation.
So, what happened to Page Rank and is it still in use? Google’s new algorithm, as mentioned earlier, uses many different factors now to determine the ranks of pages across the web. And still among them sits this old but true algorithm. No matter how Google may change, until the next trillion dollar algorithm is found that not only does and improves upon what Page Rank already does, and is impervious to manipulation, this is an algorithm that is here to stay. And we can all agree that we owe it for its work in making the internet a more accessible place for us all.
24 years now, and Page Rank is still one of the most widely used algorithms. This only goes to show the genius behind it. In fact, the algorithm is not only used for web searches. Its uses could also be found in other non-web applications all around us, ranging from sports to find the top athletes in networks of players in a team, literature to quantitatively find original writings, neuroscience, toxic waste management, and even debugging code! Clearly this algorithm hosts many rich features that speak just as much about the algorithm itself as well as the minds that came up with it. The power of any network is only as great as the meaning that is able to be extracted from it. And it is no doubt that Larry and Sergey have unlocked one of the most remarkable ways of looking at the world of networks, cementing themselves through their company as masters of information networks.
References:
https://www.link-assistant.com/news/google-page-rank-2019.html
https://blogs.cornell.edu/info2040/2014/11/03/more-than-just-a-web-search-algorithm-googles-pagerank-in-non-internet-contexts/