In thinking about social and information networks I explored less mainstream (but still well known) organizations and names such as Bing and Pinterest. I wanted to explore what differentiated various media giants in the world. In addition, why would advertisers even consider paying for less popular sites (other than reduced cost and targeted audience)? Eventually I stumbled upon a well-written paper published by Twitter themselves.
http://users.umiacs.umd.edu/~jimmylin/publications/Meyers_etal_WWW2014.pdf
I found out that not only are each of the most common internet platforms unique, but their uniqueness can be illustrated in the powerful quantities that we have already learned in class. So pay attention, as I’m about to illustrate what fundamental ideas in class were used to produce useful information for a social media platform.
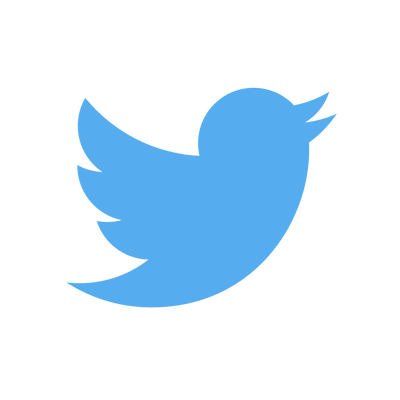
Social vs. Information Network
So first, let’s get the scope of this experiment. Twitter analyzed over 173 million active users and their follow graph for the second half of 2012. There were 20 billion edges, 42% of which were reciprocated followings. Due to the sheer size of the graph, manual computation was not feasible and so they used approximations such as the HyperANF algorithm and HyperLogLog Counter. This was all done on Twitter’s Hadoop analytics stack using Pig. They wanted to determine what kind of a network Twitter was and what were its unique features?
Perhaps most surprisingly the largest strongly connected component in Twitter was 68.7% of all vertices – a jarring gap from Facebook and MSN’s +99%. This mean that many nodes were not strongly connected into the largest SCC. We can also see a significant decrease in clustering coefficients when compared with other social media (for vertices of degree 5, 20 & 100 respectively):
- Twitter: 0.23, 0.19 & 0.14
- Facebook: 0.4, 0.3 & 0.14
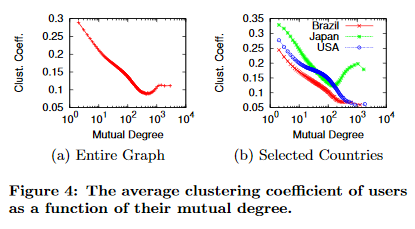
Many of these facts provide evidence that Twitter does not have characteristics that many social media platforms have. They instead point to the idea that Twitter is an information network. In fact most of the first people that users follow have higher inbound degrees. Simply put: many twitter users first come onto the platform to keep up with popular figures that interest them.
A Hybrid Platform
But Surely Twitter is still social media right? While there is no definition of a social media platform, the answer is still almost surely yes. Twitter still exhibits other traits that are indeed those of a social media platform. For example the shortest path length in Twitter is 4.05 (4.17 for only mutual followings), whereas Facebook resides with 4.74. If you also kept your eye on clustering coefficients you may have noticed that as users follow more people, the clustering coefficient becomes much more like that of Facebook’s. It is hypothesized that this because once users are more acquainted to the platform they also add other people who they know and form communities
Many more aspects of Twitter do show that is a social network, but more importantly the statistics show how it is unique. Twitter’s use as a hybrid (between information and social networks) show one of the many reasons why politicians and public figures would consider to post on this platform instead of the giant that is Facebook. Twitter is extraordinarily efficient at disseminating information to a wide audience in very little time.
Only Scratching The Surface
I am not talking ideas from the whole field of statistics, but only using the ideas that we’ve learned in class. For example, the paper also found that the in-degree distribution and mutual distribution of Twitter accounts were best fit using a power law!
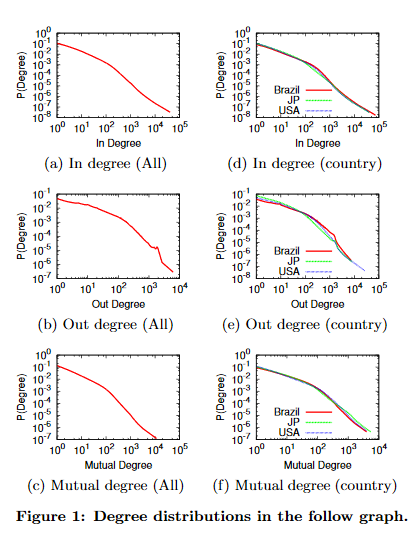
There are other factors such as two-hop neighbourhoods that demonstrate why Twitter might be better than Facebook to reach wider audiences. And if you paying attention, you might have noticed a unique quality of Japan from Figure 4. Japan’s clustering coefficients actually increase after a certain mutual degree! This may very well imply that Japan has massive cliques in their society.
Other Social Media Platforms
Clearly, other social media platforms also have their usefulness and it’d be foolish not to think of which ones any entity advertises themselves to. Facebook and Instagram may be some of the most popular platforms, but make no mistake: the others are here to stay. If you want to learn more about some statistics consider going to some of the following links:
https://www.oberlo.ca/blog/twitter-statistics
https://www.vox.com/2019/2/7/18215204/twitter-daily-active-users-dau-snapchat-q4-earnings